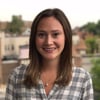
Haley Massa
Machine learning and AI have an almost mythical reputation making it hard not to view the algorithms and technologies used as having a sort of divine-like power. But as data-driven techniques continue to revolutionize the world, there has been increased interest in analyzing the unintended effects they can create.
In recent years, there have been increasing discussions about algorithmic bias in AI. These discussions have focused on how different sources of bias enter into our technology creating unintended consequences, most typically a systematically prejudiced model. Without proper protocol and testing for bias, these systemic biases can, and have, been built into models put into production.
Some real-world examples include:
- In 2015, Google was appalled to find out that their automatic image labeling system recognized a black couple as gorillas
- Amazon had to shut down a hiring algorithm they wrote when they found that it discriminated against female candidates
- Joy Buolamwini Ted Talk on fighting algorithmic bias in facial recognition technology
Breaking Down Data Bias
The first type of bias is often referred to as the Black Box problem. When building a machine learning model, we are essentially telling it to find and recognize patterns in the data we give it. However, as models get larger and more complex, so do the patterns they are piecing together. This means that, oftentimes, researchers no longer have access to understanding the assumptions made by the models, creating a “black box”. Since 1980, many researchers have been doing work to “un-black box” the assumptions made by advanced models, with recent work focusing on visualizing the relationships between the input and outputs of deep learning models seen in Google’s initiative Explainable AI.
The second type of bias commonly found in machine learning projects is bias from the data itself or data bias. Data bias occurs when the data used to train or evaluate the machine learning model is not actually representative of the true population we are trying to model. When collecting/cleaning data, there are many different statistical fallacies that researchers can fall into that can cause misrepresentation.
Beyond a company's data collection assumptions, there is also the possibility that the data itself can be systematically biased. Advanced machine learning models require a lot of data to both train and run on, often more data than a company or researcher has the capabilities to collect themselves. So, they will turn to different third parties or open sources to gain more data, whether that is scraping the internet for textual data (and in turn learning all of the harmful opinions/rhetoric of those who post online) or partnering with different organizations whose data collection standards may be different than their own.
The last type of bias we are going to discuss is human bias. Unlike the other two types of bias discussed, this bias is not limited to data science techniques. In the past year especially, there has been a lot of research and awareness made into confronting how one’s bias can affect their choices and actions — including the choices and actions that need to be made when defining key parts of machine learning models. While parts of machine learning are incredibly technical, all stages of the machine learning pipeline require the researchers behind the algorithms to make decisions for the technology, decisions like: what data to use, where to collect it from, or how do we define success in this project? These decisions help to decide the scope and values of the project.
Our Part in Reducing Data Bias
A lot of the key decisions made in machine learning modeling are made before the algorithm runs — from selecting data sources to defining metrics for success — so it is important that these outputs are accurate of the audiences they are representing. As you can see, simply removing the human aspect of decision-making and relying solely on algorithms is not a valid solution. Instead, researchers need to continue to do the work to not only combat their own biases but also work to develop solutions that remove bias from the technology being used today
At Kargo, data is at the heart of a lot of our technology, so, we have a responsibility to our clients, publishers, and the general public to ensure that we are continuing to build safe and inclusive technology. We do this through our commitment to following best practices in our data collection, cleaning, and modeling process, as well as through partnering with AI modeling organizations, like IBM Watson, that are committed to responsible development. Additionally, we stay ahead of all forthcoming data privacy and protection laws, to ensure that we are in compliance with both our publishers and advertisers.
Our data-driven approach to advertising prioritizes contextual targeting over third-party cookies. Through this approach, we are able to obtain a deeper understanding of the reader, which enables us to design more relevant ads — ultimately allowing us to create a more personalized experience overall.
To learn more about Kargo’s data collection methods, reach out.